Neural Refinement for Absolute Pose Regression with Feature Synthesis
CVPR 2024
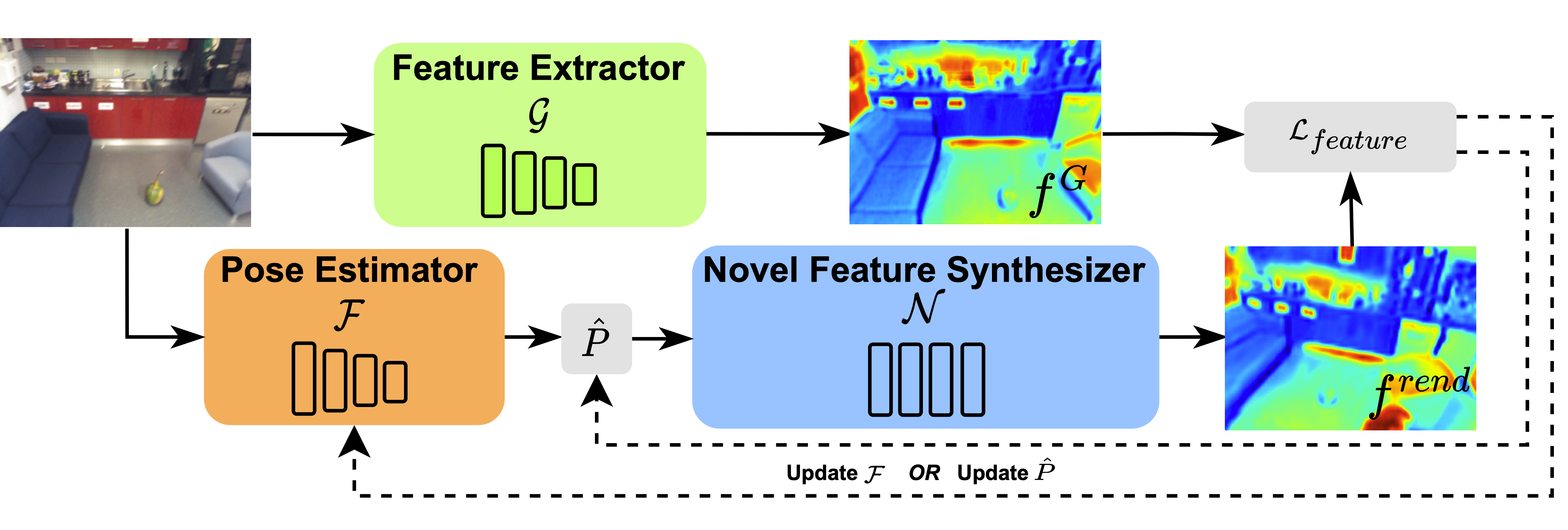
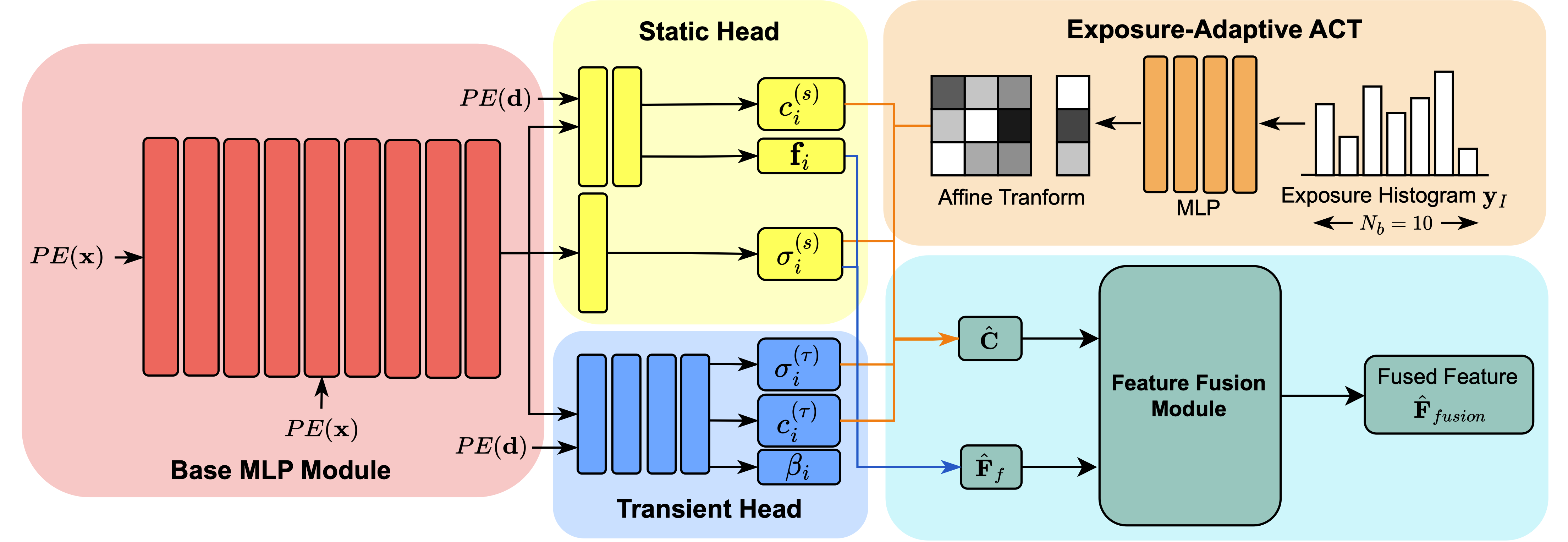
Abstract
Absolute Pose Regression (APR) methods use deep neural networks to directly regress camera poses from RGB images. Despite their advantages in inference speed and simplicity, these methods still fall short of the accuracy achieved by geometry-based techniques. To address this issue, we propose a new model called the Neural Feature Synthesizer (NeFeS). Our approach encodes 3D geometric features during training and renders dense novel view features at test time to refine estimated camera poses from arbitrary APR methods. Unlike previous APR works that require additional unlabeled training data, our method leverages implicit geometric constraints during test time using a robust feature field. To enhance the robustness of our NeFeS network, we introduce a feature fusion module and a progressive training strategy. Our proposed method improves the state-of-the-art single-image APR accuracy by as much as 54.9% on indoor and outdoor benchmark datasets without additional time-consuming unlabeled data training.
High-level Concept
Pose Refinement
BibTeX
@InProceedings{Chen_2024_CVPR,
author = {Chen, Shuai and Bhalgat, Yash and Li, Xinghui and Bian, Jia-Wang and Li, Kejie and Wang, Zirui and Prisacariu, Victor Adrian},
title = {Neural Refinement for Absolute Pose Regression with Feature Synthesis},
booktitle = {Proceedings of the IEEE/CVF Conference on Computer Vision and Pattern Recognition (CVPR)},
month = {June},
year = {2024},
pages = {20987-20996}
}